Mark Tech – Cloud-based machine learning is transforming how organizations operate, but is it the right move for you?
While it offers remarkable scalability and access to advanced tools, there are challenges, including data security issues and the risk of vendor lock-in.
Understanding the pros and cons is key to shaping your ML strategy.
We’ll explore the benefits like enhanced flexibility and reduced infrastructure costs, alongside potential drawbacks such as compliance hurdles and dependency on service providers.
As you evaluate your options, consider how these factors align with your business goals.
In the fast-paced world of cloud ML, making informed decisions is crucial for achieving success.
Key Takeaways
- Cloud-based ML offers scalability and flexibility, allowing resource adjustment based on project demands.
- Cost-effectiveness is achieved through elimination of on-premises hardware and pay-as-you-go pricing models.
- Advanced tools and resources, including cutting-edge software platforms and pre-trained model libraries, are readily available.
- Data security concerns exist, including risks of data breaches and issues with third-party access.
- Dependency on internet connectivity can impact system reliability and performance in cloud-based ML implementations.
Scalability and Flexibility
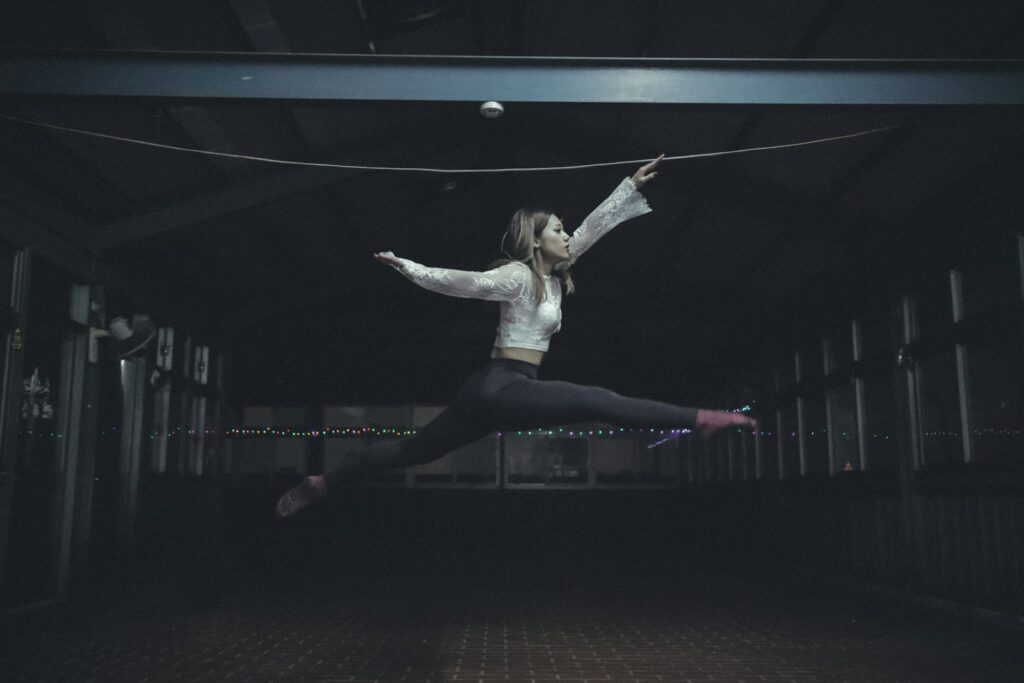
With cloud-based machine learning, you’ll find unparalleled scalability and flexibility. You can easily adjust your computational resources based on project demands, scaling up during intensive training phases and scaling down when requirements decrease. This elasticity allows you to optimize costs and efficiently manage your machine learning workloads.
Cloud platforms offer a wide array of machine learning frameworks, enabling you to choose the tools that best suit your needs. You’ll have access to pre-configured environments and the latest technologies without the hassle of maintaining on-premises infrastructure. This flexibility empowers you to experiment with different approaches and quickly iterate on your models.
However, cloud migration challenges can arise when shifting your machine learning projects to the cloud. You’ll need to address data transfer bottlenecks, guarantee proper security measures, and adapt your workflows to cloud-native architectures.
Despite these hurdles, the benefits of cloud-based machine learning often outweigh the drawbacks. You’ll gain the ability to collaborate seamlessly with distributed teams, leverage powerful GPU resources on-demand, and take advantage of managed services that simplify the deployment and monitoring of your models.
Cost-Effectiveness
Weighing the cost-effectiveness of cloud-based machine learning reveals significant advantages.
You’ll find substantial cost savings by utilizing cloud platforms, as they eliminate the need for expensive on-premises hardware and maintenance. This approach allows for better budget management, enabling you to allocate resources more efficiently and scale your ML projects as needed.
Cloud providers offer various pricing models, giving you flexibility in expense tracking and financial forecasting. You can choose pay-as-you-go options or reserved instances, depending on your project requirements and usage patterns.
This adaptability helps optimize your investment analysis and improve return on investment.
However, it’s essential to monitor your resource allocation carefully. Costs can escalate quickly if you’re not vigilant, especially with complex ML models that require extensive compute power.
To maximize cost-effectiveness, you’ll need to implement strategies for optimizing your cloud usage, such as shutting down unused instances and leveraging spot instances for non-critical tasks.
Accessibility and Collaboration
How does cloud-based machine learning revolutionize accessibility and collaboration?
Cloud platforms offer user-friendly interfaces that facilitate professionals with diverse skill sets to work together seamlessly. You’ll find that real-time collaboration becomes effortless, allowing team members to contribute simultaneously regardless of their location.
Cloud-based machine learning provides remote accessibility, empowering you to manage projects from anywhere with an internet connection. You can leverage project management tools specifically designed for ML workflows, streamlining your team’s productivity.
Additionally, cloud training resources offer continuous learning opportunities, keeping you updated with the latest ML techniques and best practices.
You’ll benefit from community support networks, where you can exchange ideas, troubleshoot issues, and learn from peers in the field. Cross-platform integration guarantees that you can work across various devices and operating systems without compatibility concerns.
This flexibility enhances your ability to collaborate with team members using different hardware or software preferences.
Advanced Tools and Resources
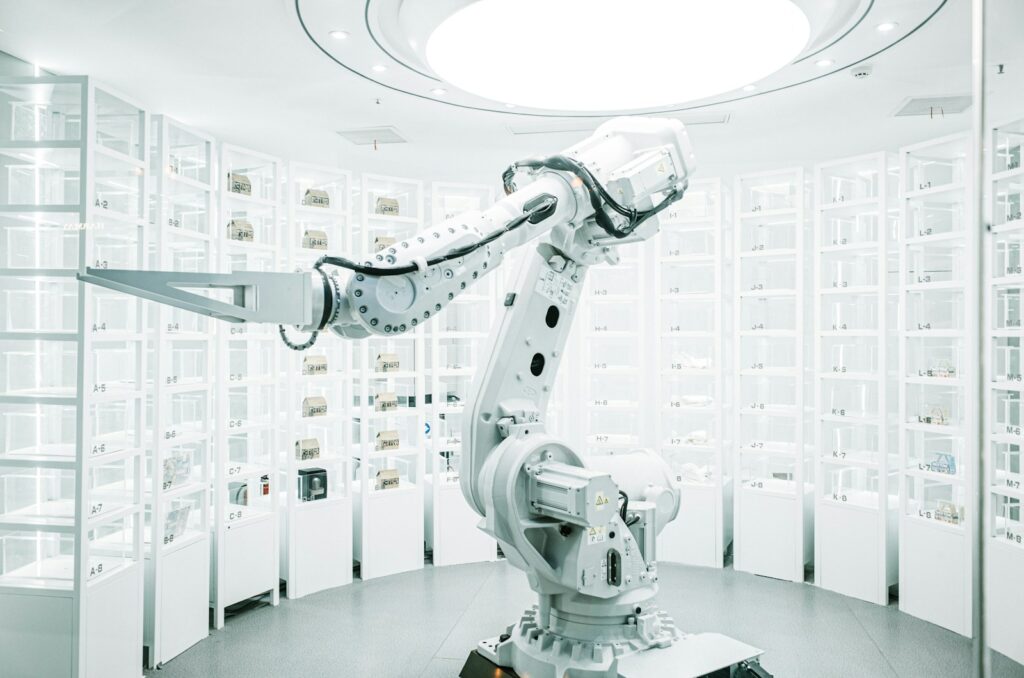
When you leverage cloud-based machine learning, you’ll gain access to cutting-edge software platforms that simplify model development and deployment.
You’ll also benefit from scalable computing resources, allowing you to train complex models without investing in expensive hardware.
Additionally, you can tap into extensive libraries of pre-trained models, accelerating your project timelines and improving overall accuracy.
Cutting-Edge Software Platforms
Cloud-based machine learning platforms are revolutionizing the way data scientists and developers approach complex AI projects.
These cutting-edge software platforms offer a suite of innovative frameworks and tools that enable you to harness the power of advanced algorithms and emerging technologies.
You’ll find extensive solutions for data preprocessing techniques, model training, and deployment strategies, all within a unified environment.
Many platforms now feature real-time analytics capabilities, allowing you to gain instant insights from your data streams.
You’ll also benefit from seamless integration tools that connect your machine learning models with existing enterprise systems.
As you explore these platforms, you’ll notice a strong emphasis on user experience, making it easier for both novices and experts to navigate complex AI workflows.
Scalable Computing Resources
Scalable computing resources form the backbone of cloud-based machine learning platforms. They enable you to efficiently manage your workload and optimize performance as your data processing needs fluctuate.
With cloud infrastructure, you can easily scale up or down your computing power, storage, and memory to match your project requirements.
Cloud providers offer advanced resource allocation tools that automatically adjust to your machine learning workflows. This dynamic scaling guarantees you’re not overpaying for idle resources or suffering from performance bottlenecks during peak demand.
You’ll find that most cloud platforms seamlessly integrate popular machine learning frameworks, allowing you to leverage familiar tools while benefiting from cloud-native optimizations.
Workload management becomes more streamlined as you can distribute tasks across multiple nodes, accelerating complex computations and model training.
Cloud-based deployment strategies also simplify the process of moving your models from development to production environments.
However, it’s essential to carefully plan your system integrations to avoid potential compatibility issues or data transfer bottlenecks.
Pre-Trained Model Libraries
One of the most significant advantages of cloud-based machine learning platforms is their extensive libraries of pre-trained models. These libraries offer you access to a vast array of pre-trained models that have been developed and fine-tuned by experts in various domains.
The pre-trained benefits are numerous, including reduced development time, lower costs, and improved accuracy for many common tasks. When you’re working on a machine learning project, you can leverage these pre-trained models as a starting point, rather than building everything from scratch. This approach allows you to focus on refining and adapting the models to your specific needs, saving valuable time and resources.
Additionally, the model selection process becomes more manageable, as you can easily compare and evaluate different pre-trained models to find the best fit for your use case. Cloud providers often update and expand their model libraries, giving you access to state-of-the-art algorithms and techniques.
This constant evolution guarantees that you’re always working with cutting-edge tools, without the need to invest heavily in research and development. However, it’s important to take into account potential limitations, such as the need for customization or the risk of over-reliance on pre-trained models for unique problems.
Data Security Concerns
When you’re considering cloud-based machine learning, data security should be at the forefront of your concerns.
You’ll need to assess the potential risks of data breaches, which can compromise sensitive information and damage your organization’s reputation.
Additionally, you must carefully evaluate third-party access issues, as your data may be handled by multiple entities in the cloud ecosystem, potentially increasing vulnerability points.
Data Breach Risks
Cloud-based machine learning platforms store vast amounts of sensitive data, making them potential targets for cybercriminals. As you consider using these services, it’s vital to understand the data breach risks associated with them.
While cloud providers implement robust security measures, including advanced encryption methods, the sheer volume of data they handle increases the potential impact of a breach.
To grasp the scope of data breach risks, consider these potential consequences:
- Exposure of confidential business information
- Compromise of personal customer data
- Financial losses due to theft or ransom demands
- Damage to your company’s reputation
- Legal and regulatory compliance violations
You’ll need to assess your cloud provider’s incident response capabilities and guarantee they align with your organization’s security requirements.
It’s important to have a clear understanding of how quickly they can detect, contain, and mitigate potential breaches. Additionally, you should implement your own security measures, such as regular security audits, employee training, and access controls.
Third-Party Access Issues
Another critical aspect of cloud-based machine learning security involves third-party access issues. When you entrust your data to cloud providers, you’re potentially exposing it to various third parties who may have access to your information. This raises significant data privacy concerns and necessitates careful consideration of user consent.
To mitigate these risks, you’ll need robust access management protocols in place. Implement strict controls over who can view, modify, or use your data. Regular third-party audits can help guarantee compliance with established security measures.
Additionally, familiarize yourself with relevant compliance frameworks and conduct thorough risk assessments to identify potential vulnerabilities. Carefully review and negotiate contractual agreements with your cloud provider. Pay close attention to service agreements, especially clauses related to data handling, storage, and access.
Confirm these agreements align with your organization’s data protection policies and legal requirements. By addressing third-party access issues proactively, you can better safeguard your sensitive information and maintain control over your machine learning operations in the cloud.
Dependency on Internet Connectivity
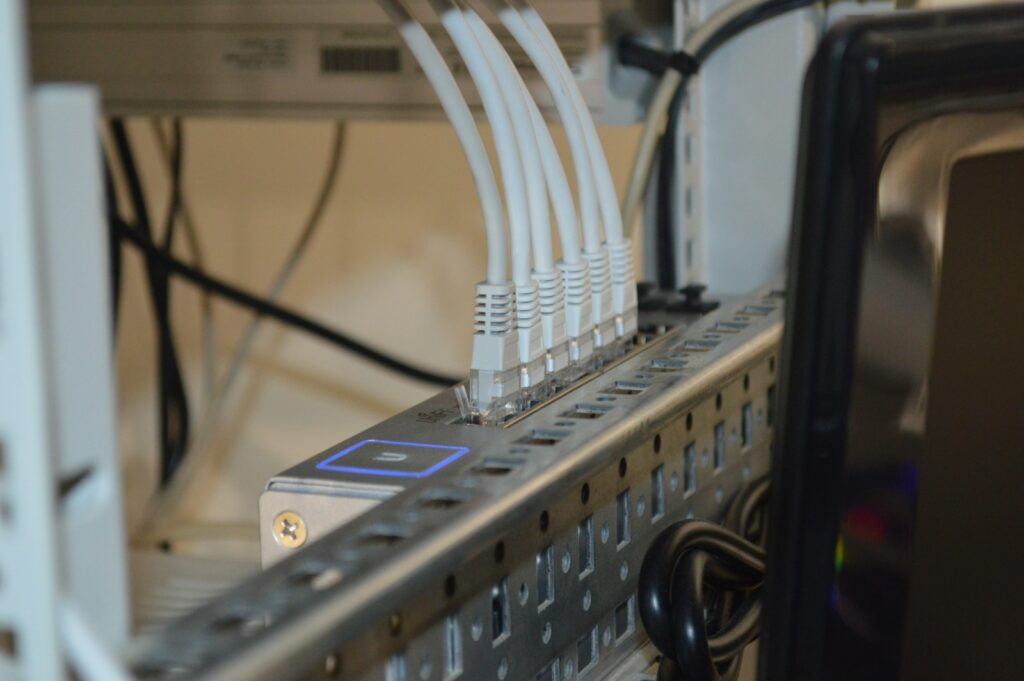
Connectivity forms the backbone of cloud-based machine learning systems. You’ll find that your reliance on internet reliability becomes paramount when utilizing cloud ML services. Connectivity challenges can greatly impact your workflow, potentially leading to service outages and disrupted operations.
Bandwidth limitations may hinder your ability to process large datasets efficiently, while latency impacts can slow down real-time predictions and model training. Remote access to your ML models and data depends entirely on a stable connection, highlighting the infrastructure dependency inherent in cloud-based solutions.
This cloud reliance means you’re vulnerable to factors beyond your control, such as:
- Internet service provider outages
- Cyber attacks on cloud infrastructure
- Natural disasters affecting data centers
- Global internet disruptions
- Local network issues
Consider developing offline capabilities to mitigate risks associated with connection instability. By implementing a hybrid approach, you can maintain some functionality during internet outages.
However, you’ll need to weigh the benefits of cloud-based ML against the potential drawbacks of connectivity-related disruptions. Ultimately, your decision should align with your organization’s tolerance for downtime and the criticality of continuous ML operations.
Vendor Lock-In
How easily can you switch between cloud providers for your machine learning needs? Vendor lock-in is a significant concern when utilizing cloud-based machine learning services. Once you’ve invested time and resources in developing models and workflows on a specific platform, shifting to another provider can be challenging and costly.
Cloud providers often use proprietary tools, APIs, and data formats, making it difficult to move your machine learning projects seamlessly. You’ll need to evaluate cloud migration strategies carefully to minimize disruption and data loss. These strategies may include containerization, using platform-agnostic tools, or adopting a multi-cloud approach.
To mitigate vendor lock-in risks, focus on portability from the start. Use open-source frameworks and standardized data formats whenever possible. Implement a modular architecture that separates core functionality from provider-specific features.
Additionally, examine vendor negotiation tactics to secure more favorable terms and exit clauses in your contracts.
While vendor lock-in can’t be entirely avoided, being aware of its implications and planning accordingly can help you maintain flexibility and control over your machine learning projects in the cloud.
Regularly assess your provider’s offerings against competitors to verify you’re getting the best value and capabilities for your needs.
Performance and Latency Issues
Cloud-based machine learning can experience performance bottlenecks and latency issues that impact your model’s efficiency and user experience.
When you’re running complex algorithms on remote servers, network bottlenecks and integration challenges can considerably affect response time. To mitigate these issues, you’ll need to focus on latency optimization and performance monitoring.
Resource allocation plays an essential role in maintaining workflow efficiency. You’ll want to implement load balancing strategies and conduct regular throughput analysis to guarantee peak performance.
Algorithm tuning is vital for improving processing speed and reducing computational demands.
Here are key performance considerations for cloud-based machine learning:
- Network latency between your local system and cloud servers
- Data transfer speeds for large datasets
- Processing power and GPU availability in the cloud
- Scalability of resources during peak usage periods
- Integration with existing systems and APIs
Compliance and Regulatory Challenges
When considering machine learning in the cloud, you’ll face significant compliance and regulatory challenges.
You must address data sovereignty concerns, ensuring your data storage and processing adhere to local laws.
Additionally, you’ll need to navigate industry-specific regulations and manage cross-border data transfers carefully to maintain compliance.
Data Sovereignty Concerns
Maneuvering the complex landscape of data sovereignty presents a considerable challenge for organizations adopting cloud-based machine learning solutions.
You’ll need to take into account where your data is stored, processed, and managed, as different countries have varying laws regarding data protection and privacy. This can greatly impact your machine learning projects, especially when dealing with sensitive information or operating across multiple jurisdictions.
To address data sovereignty concerns, you might need to implement data localization strategies. These guarantee that your data remains within specific geographic boundaries, complying with local regulations.
However, this approach can limit your ability to leverage global cloud resources and potentially affect the performance of your machine learning models.
Reflect on these key aspects of data sovereignty implications:
- Legal compliance requirements in different regions
- Restrictions on cross-border data transfers
- Potential conflicts between local laws and global data practices
- Impact on data accessibility and processing speed
- Increased complexity in managing distributed data sets
Industry-Specific Regulations Compliance
Beyond general data sovereignty issues, industry-specific regulations add another layer of complexity to cloud-based machine learning implementations. You’ll need to navigate a maze of regulatory frameworks, guaranteeing your ML models and data processing comply with industry standards. For instance, healthcare organizations must adhere to HIPAA, while financial institutions face GDPR and PCI DSS compliance.
Implementing cloud-based ML solutions requires a thorough understanding of these regulations’ legal implications. You’ll need to conduct regular compliance audits and maintain detailed audit trails to demonstrate adherence. This can be challenging when dealing with complex ML algorithms and vast datasets.
Risk management becomes essential as you balance innovation with regulatory requirements. You must consider ethical considerations, such as bias in ML models and the potential impact on individuals’ data privacy. Failure to comply can result in hefty fines and reputational damage.
However, cloud providers often offer compliance-focused tools and services to help you meet industry-specific regulations. These can simplify the process of maintaining compliance, though you’ll still need to actively manage and monitor your ML implementations to guarantee ongoing adherence to evolving regulatory landscapes.
Cross-Border Data Transfers
Cross-border data transfers present a notable hurdle for cloud-based machine learning implementations. When you’re working with global datasets or serving international customers, you’ll need to maneuver through complex legal frameworks and jurisdiction issues.
Data localization laws in some countries may require you to keep certain information within specific geographic boundaries, complicating your cloud infrastructure.
International regulations like GDPR and CCPA have far-reaching implications for data privacy and transfer protocols. You’ll need to guarantee your ML operations comply with these rules, which can vary markedly across regions.
Consider the following challenges:
- Varying data ownership laws in different countries
- Conflicting privacy regulations between jurisdictions
- Potential security risks during cross-border data transfers
- Compliance with multiple international data protection standards
- Maneuvering through complex cross-border agreements for data sharing
To address these issues, you’ll need to implement robust transfer protocols and stay updated on evolving legal frameworks.
It’s essential to work closely with legal experts to develop compliant data handling practices. Consider using regional cloud providers or implementing data residency solutions to meet localization requirements.
Control Over Infrastructure
One significant consideration when using machine learning in the cloud is the level of control you have over the infrastructure. When you opt for cloud-based ML solutions, you’re fundamentally outsourcing much of your infrastructure management to the cloud provider. This can be a double-edged sword.
On the positive side, you’re freed from many maintenance responsibilities. The cloud provider handles hardware upgrades, security patches, and system updates. This allows your team to focus on developing and improving ML models rather than worrying about the underlying infrastructure. Additionally, cloud providers often offer sophisticated tools for resource allocation, enabling you to scale your computing power up or down as needed.
However, this convenience comes at the cost of reduced control. You might face limitations in customizing the hardware or software stack to your specific needs. The control balance shifts towards the cloud provider, which can be problematic if you require highly specialized configurations.
You’re also dependent on the provider’s reliability and uptime. If they experience issues, your ML operations could be affected. Ultimately, you’ll need to weigh the benefits of reduced infrastructure management against the potential drawbacks of limited control.
Conclusion
You’ve seen the key pros and cons of cloud-based machine learning. It’s up to you to weigh these factors for your organization’s needs. Remember, scalability and advanced tools are enticing, but don’t overlook security and compliance concerns. Consider your long-term goals and data protection requirements. By carefully evaluating these aspects, you’ll make an informed decision on whether cloud-based ML is right for you. Stay adaptable and keep reassessing as technology evolves.