In 2024, cloud services are at the forefront of machine learning innovation. With platforms like Google Cloud AI, AWS SageMaker, and Microsoft Azure ML, developers have access to powerful tools that simplify the creation and deployment of AI models.
However, selecting the right service hinges on your unique requirements and objectives.
Whether you’re part of a startup or a large enterprise, the choice you make can significantly influence the outcome of your projects. Key factors to keep in mind include pricing, scalability, ease of use, and the range of integrated tools.
Each platform offers distinct advantages, so aligning their features with your specific goals is essential.
By focusing on what matters most to your team and your projects, you can make a well-informed decision that propels your machine learning initiatives forward.
Key Takeaways
- Google Cloud AI Platform offers extensive ML lifecycle support and competitive pricing for large-scale tasks.
- AWS SageMaker provides a fully managed service with pre-built algorithms and seamless integration with other AWS services.
- Microsoft Azure Machine Learning features automated ML capabilities and end-to-end lifecycle management.
- Databricks Unified Data Analytics excels in Apache Spark-based analytics and offers collaborative notebooks for team projects.
- IBM Watson Studio includes AutoAI for automated model building and fosters collaboration among data scientists in enterprise settings.
Top Machine Learning Cloud Platforms
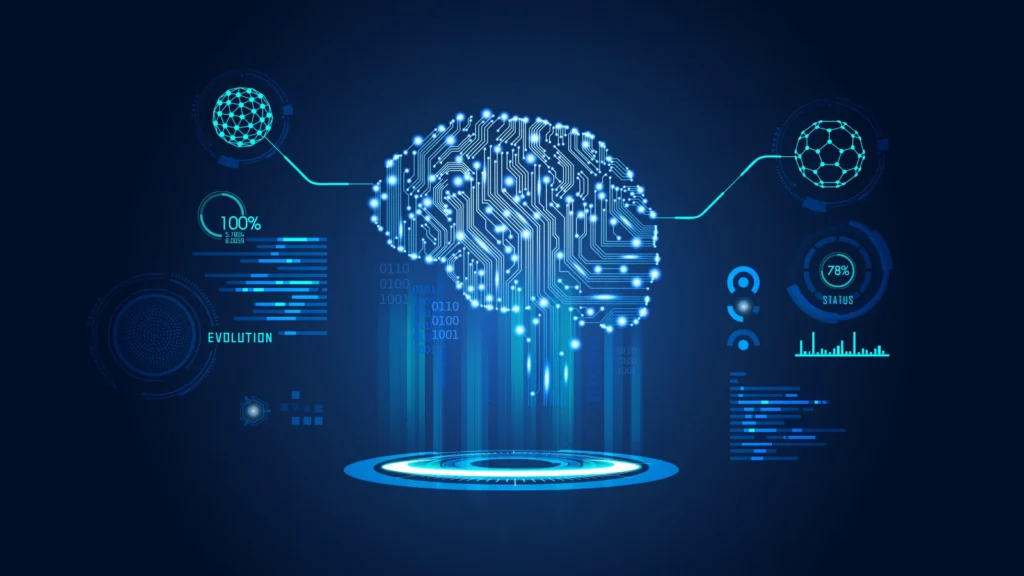
The landscape of machine learning cloud platforms is rich with options, each offering unique features to suit different needs. Google Cloud AI Platform stands out for its extensive support of the entire machine learning lifecycle and competitive pricing, making it ideal for large-scale tasks.
If you’re looking for a fully managed service with pre-built algorithms and easy deployment, AWS SageMaker might be your best bet. It offers built-in Jupyter notebooks and seamless AWS integration.
Microsoft Azure Machine Learning provides end-to-end ML lifecycle management and automated machine learning capabilities, perfect for businesses leveraging Azure DevOps.
For those working with big data, Databricks Unified Data Analytics excels in Apache Spark-based analytics and offers collaborative notebooks with various data source integrations.
IBM Watson Studio is a strong contender for enterprises, featuring AutoAI for automated model building and fostering collaboration among data scientists.
When choosing a cloud platform for your machine learning needs, consider factors like integration with existing systems, pricing models, and specific features that align with your project requirements.
Each of these top platforms offers unique strengths, so evaluate them based on your team’s expertise and project goals.
Key Features to Consider
Selecting the right cloud platform for machine learning hinges on identifying key features that align with your project’s needs.
You’ll want to prioritize high-performance computing resources that support distributed training and hyperparameter tuning, especially when working with large datasets and complex models. Look for cloud GPU platforms that offer the computational power necessary for intensive tasks.
Ensure the platform provides compatibility with programming languages and frameworks you’re familiar with, such as TensorFlow, PyTorch, and Scikit-learn. This flexibility allows you to work in your preferred environment.
Consider platforms with built-in model development tools that streamline the entire machine learning lifecycle, from data preparation to deployment.
Don’t overlook collaboration features like shared workspaces and version control, which are vital for team-based projects. These tools enhance communication and project management among team members.
Additionally, assess the platform’s data visualization capabilities, as they’re essential for interpreting model performance and gaining insights from your data.
Pricing Models and Costs
Cost considerations play an essential role in choosing cloud services for machine learning. When evaluating pricing models, you’ll find that major cloud providers offer competitive pricing structures tailored to different usage patterns.
Google Cloud Platform entices new users with a generous $300 credit for the first 90 days, allowing you to explore their services before committing. Usage charges start at $0.19/hour, making it an attractive option for those just starting out.
AWS SageMaker adopts a pay-as-you-go model, ensuring you’re only billed for the resources you actually use. This flexibility can be particularly beneficial if your usage fluctuates.
Azure’s consumption-based pricing covers compute, storage, and data transfer costs. Their pricing calculator helps you estimate expenses based on your specific needs.
For those seeking a more structured approach, DataRobot offers subscription pricing that scales with your usage. This model includes additional costs for advanced features and monitoring services.
Oracle AI Platform Cloud Service implements tiered pricing based on service levels and resource utilization. They also provide reserved instances, which can reduce costs for consistent usage patterns.
Industry-Specific Solutions
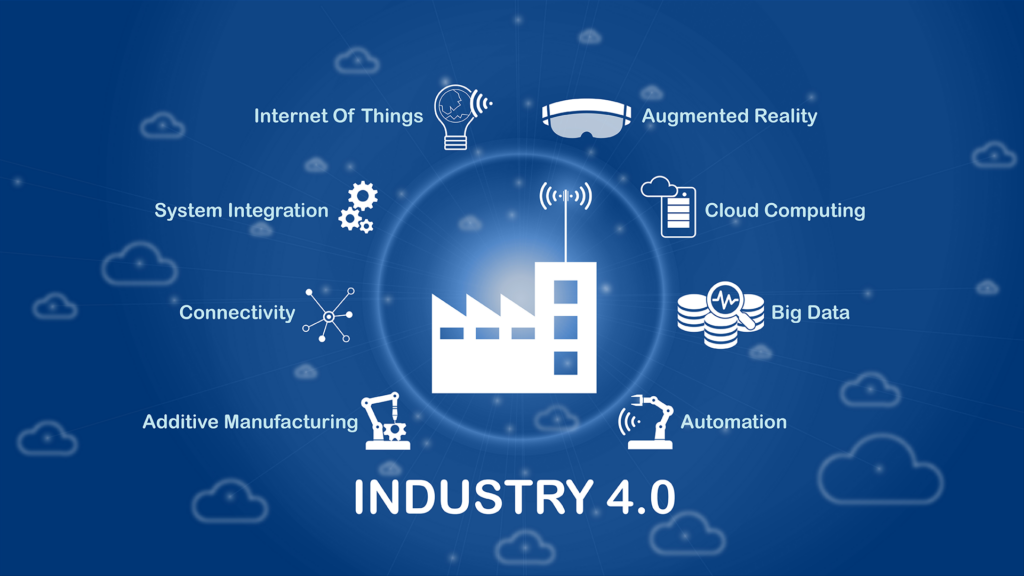
Over the past decade, cloud-based machine learning solutions have evolved to address industry-specific challenges. You’ll find AI applications tailored to various sectors, offering powerful tools for data analysis and predictive models.
In healthcare, platforms like IBM Watson Health leverage vast medical data to provide AI-driven insights for patient care and operational efficiencies.
Financial institutions benefit from services such as AWS SageMaker and Microsoft Azure, which enable automated fraud detection and risk assessment through advanced predictive modeling.
For retail, Google Cloud AI Platform supports personalization efforts and inventory management, utilizing machine learning for demand forecasting.
In manufacturing, Databricks Unified Data Analytics offers solutions for predictive maintenance and supply chain optimization, analyzing sensor data to reduce downtime.
Marketing teams can leverage automated machine learning tools like DataRobot to analyze consumer behavior and optimize campaigns through targeted audience segmentation.
These platforms enable you to create sophisticated predictive models without extensive coding knowledge.
Integration and Scalability Options
When considering cloud services for machine learning, integration and scalability are essential factors. Leading platforms like AWS SageMaker, Google Cloud AI Platform, and Azure Machine Learning offer robust solutions to meet these needs. These services provide seamless integration with popular data sources and tools, enhancing your data ingestion and model training processes.
Scalability is a key strength of these platforms, allowing you to easily adjust computational resources as your workloads and user demands grow. This flexibility guarantees you’re not overpaying for unused capacity or struggling with limited resources during peak times.
Here are some notable integration and scalability features:
- AWS SageMaker’s built-in support for multiple frameworks like TensorFlow and PyTorch
- Google Cloud AI Platform’s integration with BigQuery for efficient data analysis
- Azure Machine Learning’s smooth integration with Azure DevOps for streamlined project management
- Databricks Unified Data Analytics’ collaboration features for easy sharing of models and data
These platforms offer various deployment options, enabling you to choose the best fit for your project.
Whether you’re working on small-scale experiments or large-scale production models, these cloud services provide the tools and flexibility you need to succeed in your machine learning endeavors.
Conclusion
You’ve got a wealth of options for cloud-based machine learning in 2024. Whether you’re drawn to Google Cloud’s power, AWS’s managed environment, or Azure’s lifecycle management, you’ll find a platform that fits your needs. Don’t forget to weigh features, pricing, and scalability against your specific requirements. As you explore these services, you’ll reveal new possibilities for your ML projects, streamlining development and deployment across various industries.