Mark Tech – Cloud AI tools are transforming the way data scientists work, offering innovative solutions to enhance productivity and streamline processes.
With an array of platforms available, such as Google Cloud AI Platform and Cloudera Machine Learning, it’s essential to identify which tools can elevate your projects.
In this guide, we’ll spotlight ten cloud AI tools that stand out for their unique features and capabilities.
By understanding the strengths of each option, you can harness their power to drive innovation in your projects.
Let’s explore these game-changing tools and how they can redefine your approach to data science.
Key Takeaways
- Integrated Machine Learning Platforms like Google Cloud AI Platform and Amazon SageMaker offer comprehensive suites for end-to-end AI workflows.
- Automated Machine Learning Solutions such as DataRobot and H2O.ai streamline the data science process with automated feature engineering and model selection.
- Cloud-Based Analytics Platforms like Databricks provide collaborative workspaces for big data analytics and machine learning projects.
- User-Friendly Machine Learning Platforms like BigML offer accessible tools for developing predictive models without extensive programming knowledge.
- Managed Jupyter Notebooks provide powerful, customizable environments for data science projects with built-in version control and security features.
Google Cloud AI Platform
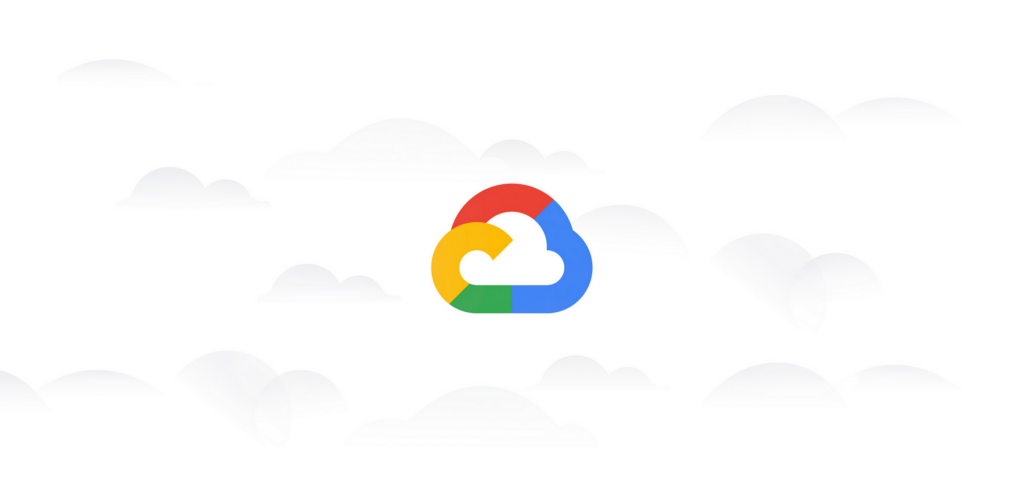
A powerhouse in the cloud AI arena, Google Cloud AI Platform offers data scientists a thorough suite of tools for machine learning and artificial intelligence.
You’ll find a detailed ecosystem that supports your entire AI workflow, from data preprocessing techniques to AI model deployment. The platform provides robust cloud data storage options, guaranteeing your datasets are secure and easily accessible.
You can leverage popular machine learning frameworks like TensorFlow and scikit-learn, streamlining your development process. For real-time analytics and predictive modeling tools, Google Cloud AI Platform offers scalable infrastructure options that adapt to your project’s needs.
As you develop and deploy models, you’ll appreciate the platform’s model monitoring solutions, which help maintain performance and accuracy over time. Google also prioritizes AI ethics considerations, providing resources and guidelines to guarantee responsible AI development.
Cloud security measures are integral to the platform, safeguarding your data and models. Whether you’re working on small-scale projects or enterprise-level applications, you’ll find the flexibility and power you need to bring your AI initiatives to life.
With Google Cloud AI Platform, you’re equipped to tackle complex data science challenges efficiently and effectively.
Amazon SageMaker
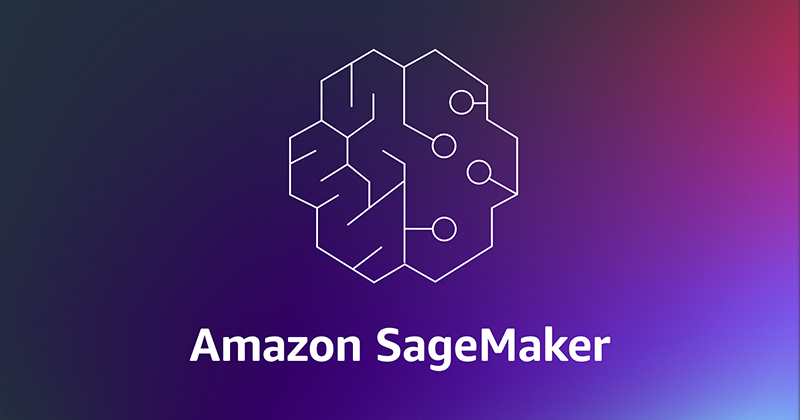
When you’re looking for a thorough machine learning platform, Amazon SageMaker offers an integrated ML workflow that streamlines your data science projects.
You’ll find tools for every stage of the ML lifecycle, from data preparation to model deployment and monitoring.
SageMaker also provides managed Jupyter Notebooks, allowing you to easily create and share documents that contain live code, equations, visualizations, and narrative text.
Integrated ML Workflow
Amazon SageMaker stands out as an all-encompassing platform for building, training, and deploying machine learning models at scale. It provides an integrated ML workflow that streamlines your entire data science process. You’ll find robust tools for data preprocessing, feature engineering, and experiment tracking, enabling you to efficiently prepare and analyze your datasets.
SageMaker’s pipeline orchestration capabilities allow you to automate complex ML workflows, ensuring consistency and reproducibility. You can easily manage version control for your models and datasets, keeping track of changes and iterations.
The platform also offers advanced model explainability features, helping you understand and interpret your models’ decisions.
When it comes to model deployment, SageMaker provides seamless options for scaling and monitoring your models in production. You can leverage its performance monitoring tools to track your models’ effectiveness and make data-driven improvements.
Data governance and cloud security features are built-in, ensuring compliance with industry standards and protecting your sensitive information.
With SageMaker’s integrated ML workflow, you’ll have everything you need to develop, deploy, and maintain high-quality machine learning models efficiently.
Managed Jupyter Notebooks
SageMaker’s managed Jupyter notebooks offer a powerful, flexible environment for your data science and machine learning projects. You’ll find a thorough suite of tools for data visualization, interactive widgets, and data preprocessing, all within a secure cloud environment.
These notebooks streamline your workflow by handling resource management and performance optimization, allowing you to focus on your analysis and model development.
With SageMaker’s managed notebooks, you’ll benefit from built-in version control capabilities, making it easy to track changes and collaborate with team members.
The platform’s environment configuration options let you customize your workspace to suit your specific needs, guaranteeing you have access to the libraries and frameworks you require. You can seamlessly share notebooks with colleagues, fostering collaboration and knowledge exchange within your organization.
Cloud security features protect your sensitive data and intellectual property, while the platform’s scalability guarantees you can handle projects of any size.
Microsoft Azure Machine Learning
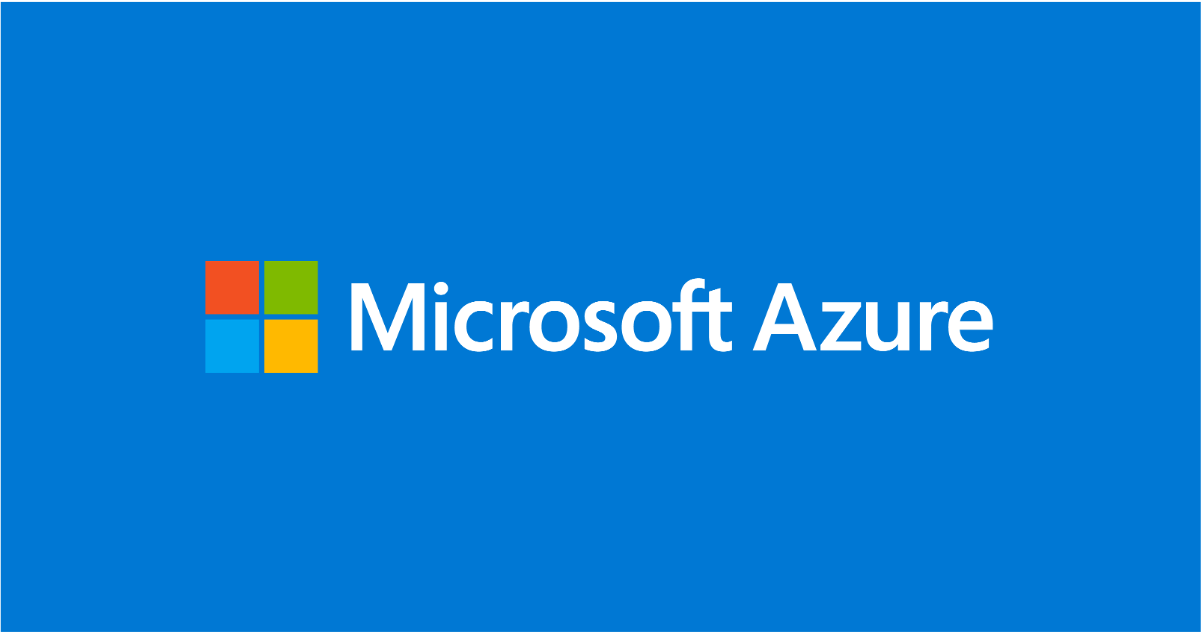
Microsoft Azure Machine Learning stands out as a powerhouse in the cloud-based AI landscape. It offers a thorough suite of tools designed to streamline your data science workflow.
With Azure ML, you’ll find robust Data Preparation features that help clean and transform your datasets efficiently. The platform’s Scalability Solutions guarantee your models can handle large-scale computations without breaking a sweat.
You’ll appreciate Azure ML’s flexible Deployment Strategies, allowing you to easily shift from development to production. The platform’s Model Interpretability tools give you insights into your AI’s decision-making process, enhancing transparency and trust.
Azure ML’s Integration Capabilities let you seamlessly connect with other Azure services and third-party tools, creating a cohesive ecosystem for your projects. Cost Management features help you optimize your resource usage and budget, while Data Governance tools guarantee compliance with regulatory requirements.
Azure ML’s Security Features protect your sensitive data and models from potential threats. For advanced users, the platform supports Custom Algorithms, enabling you to implement cutting-edge techniques tailored to your specific needs.
With Azure Machine Learning, you’re equipped to tackle complex AI challenges efficiently and effectively.
IBM Watson Studio
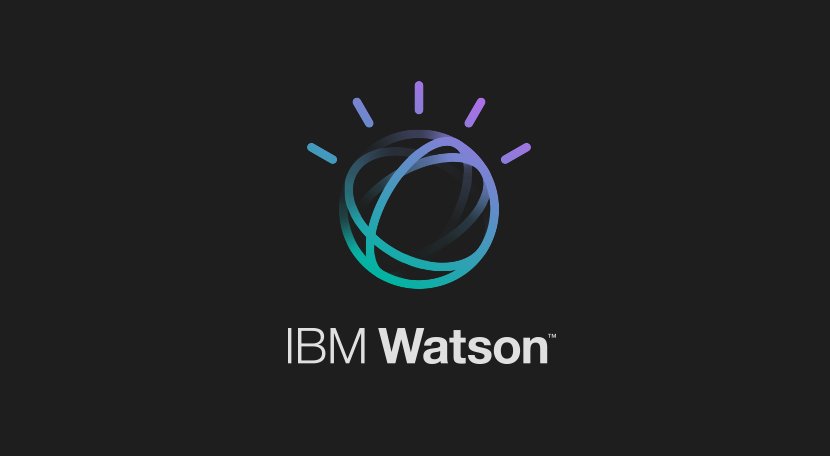
IBM Watson Studio offers you powerful tools for collaborative data analysis and automated model building.
You’ll find features that enable seamless teamwork among data scientists, developers, and domain experts.
With Watson Studio’s AutoAI capabilities, you can accelerate your machine learning workflows by automating the process of model selection and hyperparameter tuning.
Collaborative Data Analysis
Collaboration lies at the heart of IBM Watson Studio’s data analysis capabilities. You’ll find an extensive suite of tools designed to enhance team collaboration and streamline your data science projects. With Watson Studio, you can easily share data, code, and insights with your colleagues, fostering a more efficient workflow.
The platform offers interactive dashboards that allow you to visualize complex data sets and present your findings in a clear, compelling manner. You’ll appreciate the built-in version control system, which helps you track changes and manage different iterations of your work.
Project management features enable you to assign tasks, set deadlines, and monitor progress, ensuring your team stays on track. Watson Studio’s cloud storage solution provides secure access to your data from anywhere, facilitating seamless collaboration across geographical boundaries.
You can leverage real-time feedback mechanisms and communication tools to exchange ideas and receive input from team members. The platform also supports peer reviews, enabling you to maintain high-quality standards throughout your projects.
Automated Model Building
One of the standout features of IBM Watson Studio is its automated model building capability. This powerful tool streamlines your machine learning workflow, allowing you to focus on higher-level tasks.
With automated pipelines, you can efficiently handle data preprocessing, feature engineering, and model validation in a single, cohesive process.
Watson Studio’s automated model building incorporates advanced techniques like hyperparameter tuning and ensemble methods to optimize your models. You’ll find that the platform automatically selects the best-performing algorithms and fine-tunes their parameters, saving you countless hours of manual experimentation.
The tool also provides extensive performance metrics, helping you quickly assess model effectiveness.
As you work with Watson Studio, you’ll appreciate its interpretability tools, which offer insights into your model’s decision-making process. This transparency is essential for explaining results to stakeholders and ensuring compliance with regulations.
When it comes to deployment strategies, the platform offers seamless options for integrating your models into production environments. With workflow automation, you can easily replicate successful pipelines across different projects, further enhancing your productivity as a data scientist.
DataRobot

DataRobot stands out as a powerful automated machine learning platform designed to streamline the data science workflow. With its extensive set of DataRobot features, you’ll find everything from automated feature engineering to model deployment and monitoring. The platform offers seamless DataRobot integrations with popular data sources and tools, enhancing your existing workflow.
When considering DataRobot pricing, you’ll find options tailored to various business sizes and needs. DataRobot use cases span industries, from finance to healthcare, demonstrating its versatility.
However, be aware of DataRobot limitations, such as potential overfitting in complex scenarios. The platform’s robust DataRobot customer support guarantees you’ll have assistance when needed, contributing to a positive DataRobot user experience.
In DataRobot comparisons with other AutoML tools, it often ranks highly for its accuracy and ease of use. To get started, you can access numerous DataRobot tutorials, helping you quickly leverage its capabilities.
DataRobot success stories from leading companies showcase its real-world impact, boosting confidence in its effectiveness. By incorporating DataRobot into your workflow, you’ll accelerate your data science projects and drive more value from your data.
H2O.ai
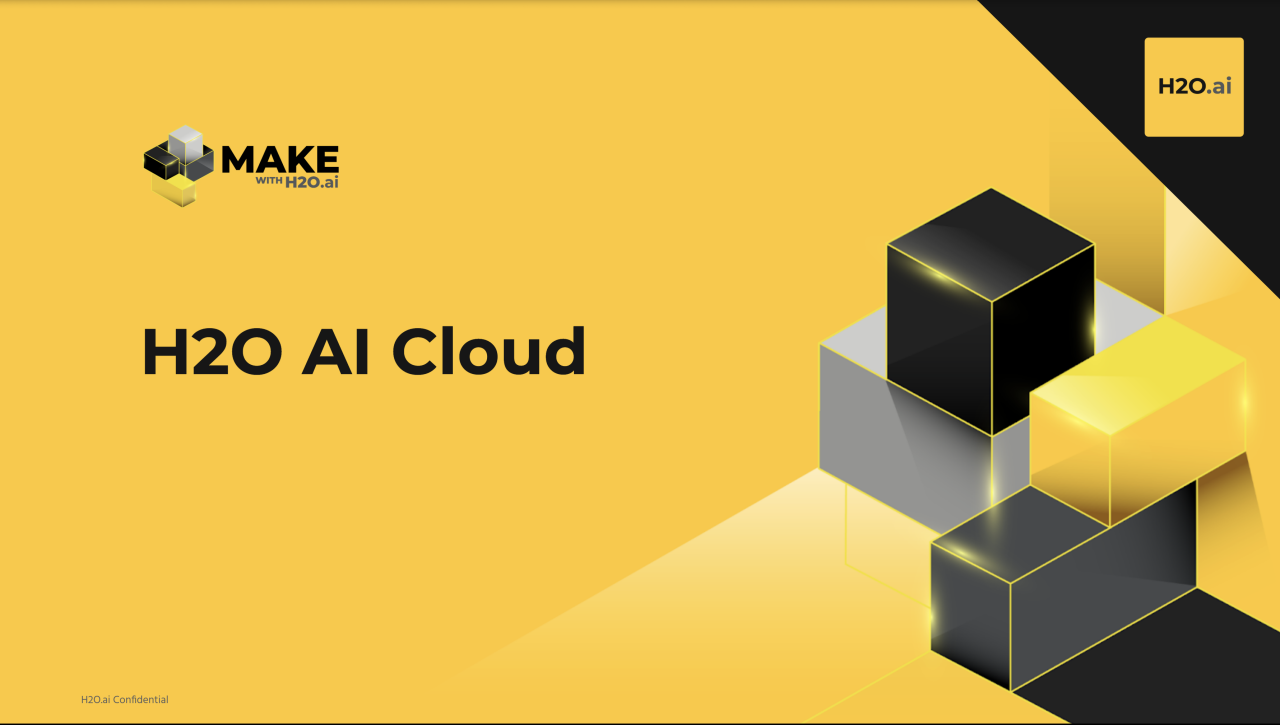
H2O.ai frequently emerges as a top choice for data scientists seeking robust, open-source machine learning solutions. This platform offers a thorough suite of AI and machine learning tools that cater to various data science needs.
You’ll find that H2O.ai features include automated machine learning, distributed computing, and deep learning capabilities, all accessible through user-friendly interfaces.
One of the platform’s standout offerings is H2O AutoML, which automates the process of building and comparing multiple models. This tool saves you time and helps identify the best-performing algorithms for your specific dataset.
Additionally, H2O.ai provides scalable implementations of popular machine learning algorithms, such as gradient boosting, random forests, and deep learning neural networks.
The h2o.ai community is another valuable aspect of the platform. You can tap into a wealth of knowledge shared by data scientists, developers, and researchers worldwide.
This community-driven approach fosters collaboration, problem-solving, and continuous improvement of the platform.
With support for multiple programming languages, including R, Python, and Java, H2O.ai integrates seamlessly into your existing workflow, making it a versatile choice for data scientists across various industries and skill levels.
TensorFlow on Cloud
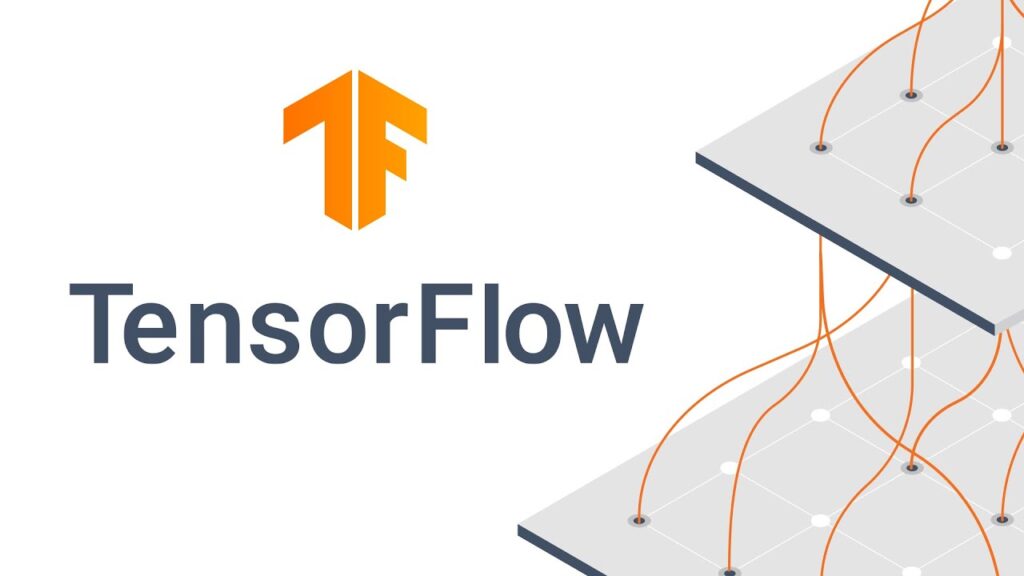
TensorFlow on Cloud represents a powerful combination of Google’s open-source machine learning framework and cloud computing resources. This integration allows you to leverage TensorFlow’s extensive features and libraries while harnessing the scalability and performance of cloud platforms.
You’ll find that TensorFlow on Cloud offers seamless deployment options, making it easier to bring your machine learning models into production environments.
When working with TensorFlow on Cloud, you’ll benefit from:
- Enhanced performance through distributed training and GPU acceleration
- Simplified model deployment using containerization technologies
- Easy integration with other cloud services for data processing and storage
TensorFlow’s compatibility with various cloud providers guarantees you’re not locked into a single platform.
You can take advantage of TensorFlow’s extensive community support, tutorials, and regular updates to stay current with the latest advancements in machine learning. The cloud environment also facilitates collaboration, allowing you to share TensorFlow models and workflows with team members more efficiently.
BigML
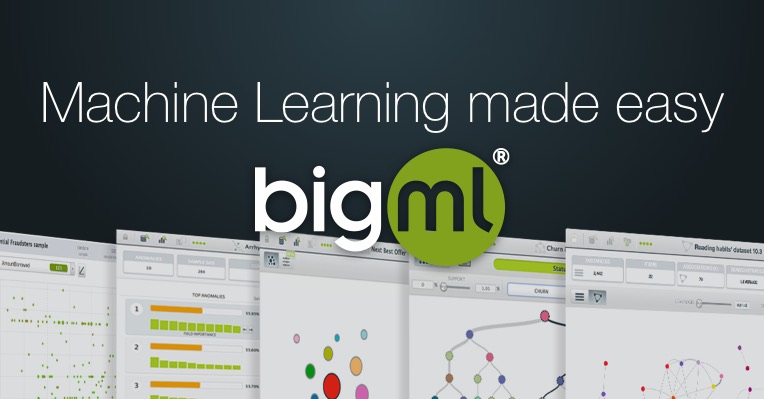
Another powerful cloud-based AI tool for data scientists is BigML, a user-friendly machine learning platform designed to simplify the process of creating and deploying predictive models.
BigML features a wide range of algorithms, including decision trees, ensembles, and deep neural networks, allowing you to tackle various machine learning tasks efficiently. Its intuitive interface makes it accessible to both beginners and experienced data scientists.
BigML use cases span industries such as finance, healthcare, and marketing, where you can leverage its capabilities for tasks like fraud detection, patient outcome prediction, and customer segmentation.
The platform offers seamless BigML integration with popular tools and APIs, enhancing your existing workflow. While BigML pricing is competitive, it’s crucial to evaluate your specific needs and budget.
Regarding BigML scalability, the platform can handle large datasets and complex models effectively. Some BigML advantages include its visualization tools and automated model optimization.
However, BigML limitations include a lack of support for certain advanced algorithms. When comparing BigML to other platforms, you’ll find it excels in ease of use but may lag in customization options.
To get started, explore BigML tutorials and engage with the active BigML community for support and best practices.
Databricks Unified Analytics Platform
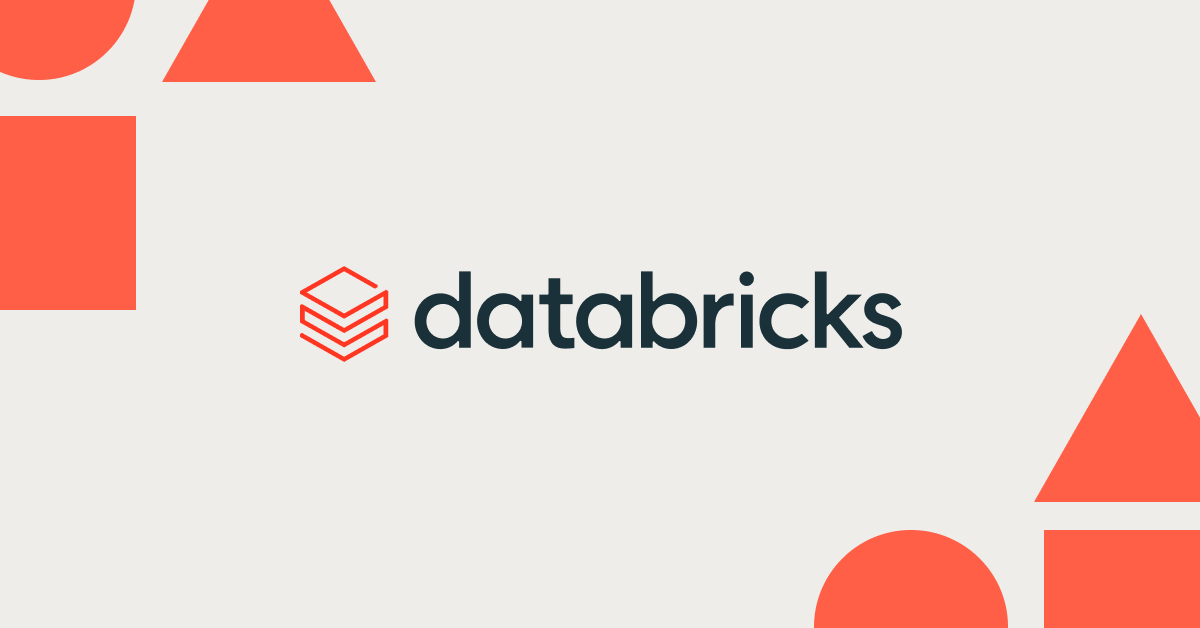
Moving from user-friendly platforms to enterprise-scale solutions, the Databricks Unified Analytics Platform stands out as an extensive cloud-based environment for big data analytics and machine learning.
It’s designed to simplify the process of building and deploying AI solutions at scale, combining the best of data engineering and data science workflows.
Databricks features a collaborative workspace where you can easily share notebooks, dashboards, and libraries with your team. It integrates seamlessly with popular cloud providers, allowing you to leverage your existing infrastructure.
One of its key components is Delta Lake, an open-source storage layer that brings reliability to data lakes.
Here are three standout capabilities of Databricks:
- MLflow integration for end-to-end machine learning lifecycle management
- Auto-scaling clusters for efficient resource utilization
- Built-in support for popular frameworks like TensorFlow, PyTorch, and Spark MLlib
You’ll find that Databricks excels in handling large-scale data processing and advanced analytics tasks.
Its unified approach to data engineering, data science, and machine learning streamlines your workflow, enabling you to focus on deriving insights and building powerful AI models.
With its robust security features and enterprise-grade performance, Databricks is well-suited for organizations looking to accelerate their AI initiatives.
Cloudera Machine Learning
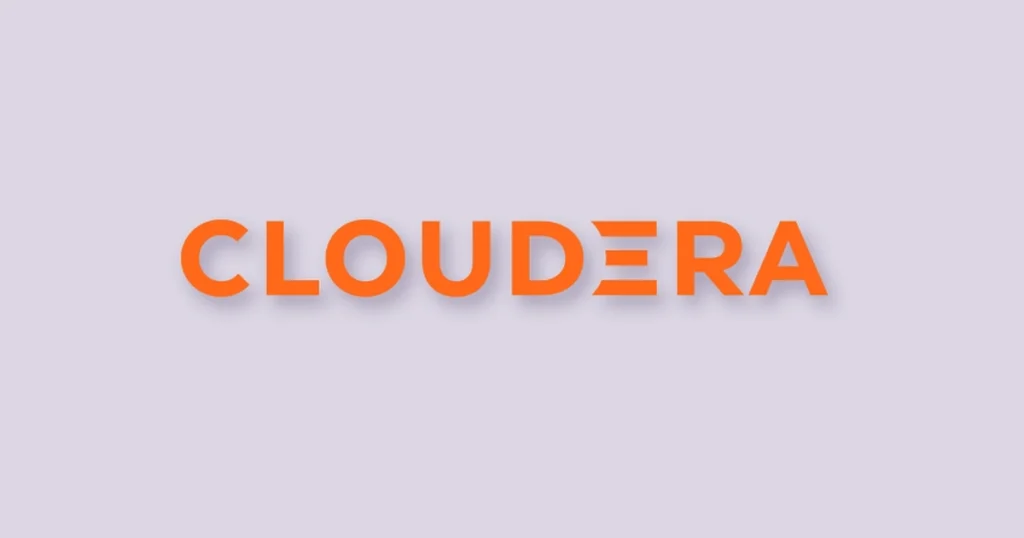
Cloudera’s powerhouse platform, Machine Learning, offers data scientists a thorough suite of tools for developing, deploying, and managing AI models at scale.
You’ll find numerous Cloudera advantages, including seamless integrations with popular data science libraries and frameworks. The platform’s robust Cloudera security features guarantee your sensitive data and models remain protected throughout the development lifecycle.
When it comes to Cloudera scalability, you can easily handle large datasets and complex models without compromising performance. The intuitive Cloudera user experience streamlines your workflow, allowing you to focus on creating innovative solutions.
Cloudera deployment options are flexible, supporting both on-premises and cloud environments.
You’ll appreciate the platform’s impressive Cloudera performance, which optimizes resource utilization and accelerates model training. Advanced Cloudera analytics capabilities enable you to gain deeper insights from your data.
If you encounter any issues, Cloudera support is readily available to assist you. Additionally, you can tap into the vibrant Cloudera community for knowledge sharing and collaboration.
With Cloudera Machine Learning, you’re equipped with a complete toolset to tackle even the most challenging AI projects efficiently and effectively.
Conclusion
You’ve now explored ten powerful cloud AI tools that’ll revolutionize your data science workflow. By leveraging these platforms, you’ll streamline your processes, collaborate more effectively, and access deeper insights from your data. Whether you’re building models, automating tasks, or visualizing results, these tools offer the flexibility and power you need. Don’t hesitate to experiment with different options to find the perfect fit for your projects. Embrace these cloud AI solutions and watch your productivity soar.