MarkTech – The world of cloud-based machine learning platforms is filled with exciting possibilities. From Google’s Vertex AI to Amazon’s SageMaker, these tools can turn your data into valuable insights.
But how do you choose the right one for your organization?
Understanding the unique features, performance capabilities, and pricing of each platform is key.
In this article, we’ll highlight the seven leading cloud-based ML platforms. Discover which one could be the perfect match for your data science projects and elevate your analytics to the next level.
Key Takeaways
- Major cloud ML platforms include Google Vertex AI, AWS SageMaker, Microsoft Azure ML, Databricks, and IBM Watson Studio.
- Key features across platforms include AutoML, collaborative tools, data visualization, and high-performance computing options.
- Pricing models vary, with pay-per-use and subscription options available; cost efficiency depends on workload and data volume.
- Integration capabilities with existing systems and support for multiple programming languages are crucial for platform selection.
- User experience and learning curve differ among platforms, with some offering more user-friendly interfaces and extensive training resources.
Key Features of ML Platforms
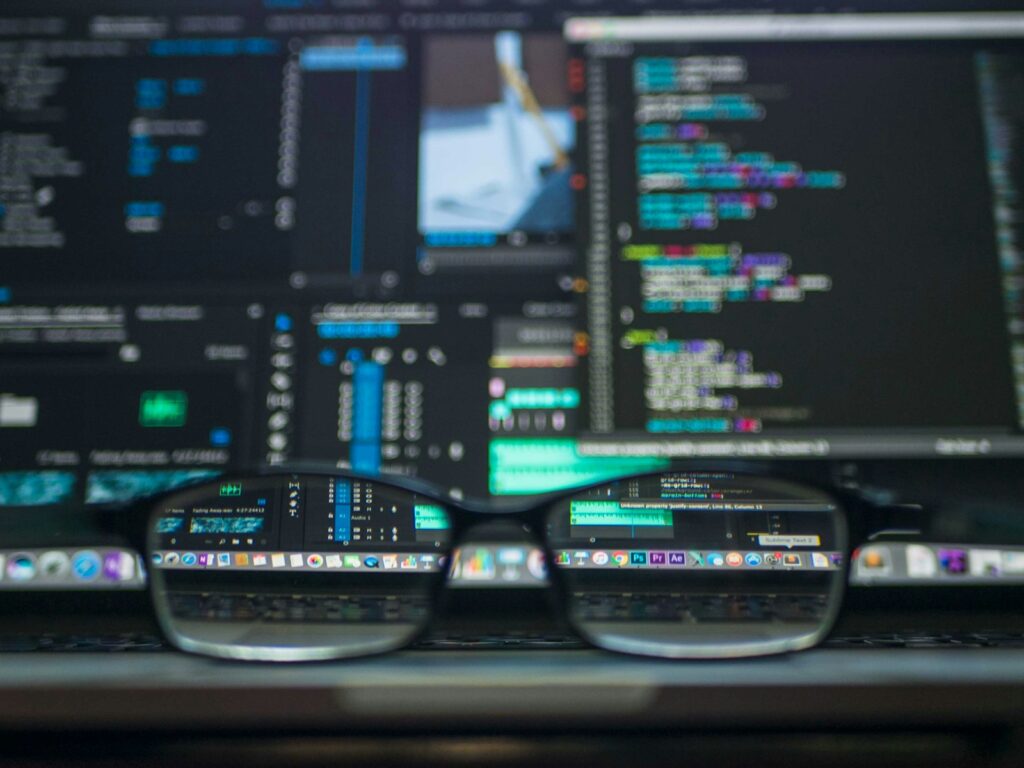
Cloud-based machine learning platforms offer an extensive suite of features designed to streamline the ML workflow. You’ll find integrated tools for model development, training, and deployment, allowing you to manage the entire ML lifecycle efficiently.
These platforms leverage cloud computing resources to provide high-performance computing options, including GPU and TPU support, enabling you to train complex models quickly and scale resources based on demand.
One of the standout features is automated machine learning (AutoML), which simplifies the model creation process for users of varying expertise. You’ll also benefit from data labeling capabilities and model versioning, ensuring your projects remain organized and well-documented.
Collaboration tools, such as shared notebooks and team-based project management, facilitate seamless teamwork among data scientists and ML engineers.
Data visualization and analytics features are built into many machine learning platforms, helping you extract insights and make informed decisions through intuitive graphical representations of model performance and data trends.
With these thorough features, cloud-based ML platforms empower you to develop, train, and deploy machine learning models efficiently, regardless of your experience level or project complexity.
Top Contenders in Cloud ML
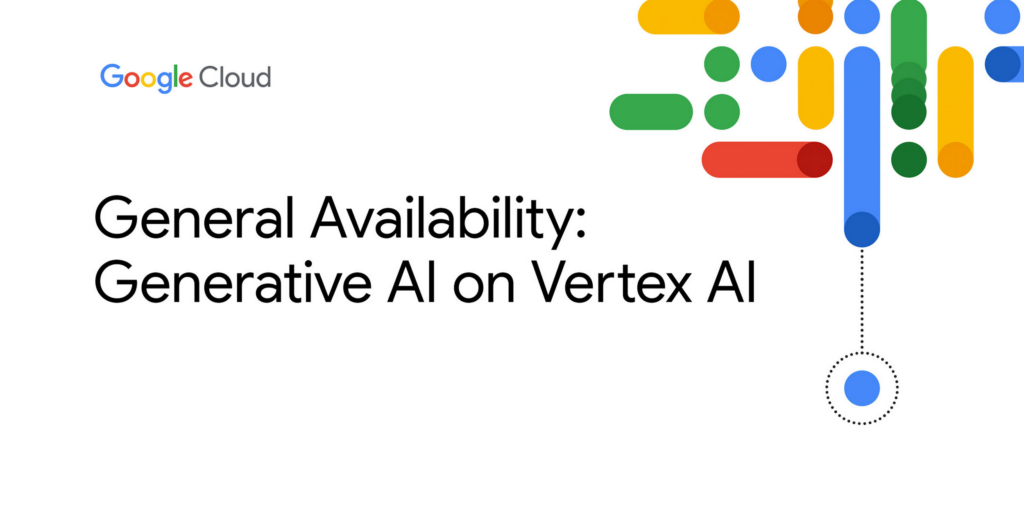
You’ll find several major players dominating the cloud-based machine learning landscape, each offering unique strengths.
When comparing key features, you’ll notice differences in areas like model deployment, integration capabilities, and specialized tools for specific industries.
As you evaluate these platforms, consider how their industry-specific ML solutions align with your organization’s needs and goals.
Leading Cloud ML Providers
Among the top contenders in cloud-based machine learning platforms, several providers stand out for their extensive offerings and innovative features. Google Vertex AI, AWS SageMaker, Microsoft Azure Machine Learning, Databricks, and IBM Watson Studio each bring unique strengths to the table, catering to different aspects of the machine learning lifecycle.
You’ll find that these cloud providers offer a range of tools for building, training, and deploying deep learning models. Google Vertex AI leverages containerized approaches with GPU capabilities, while AWS SageMaker focuses on a developer-centric platform. Microsoft Azure Machine Learning integrates seamlessly with other Microsoft products, offering drag-and-drop model design.
When choosing a platform, consider:
- Your team’s expertise and familiarity with specific ecosystems
- The complexity of your machine learning projects
- Your budget and pricing preferences
Databricks stands out for its Lakehouse architecture, ideal for large data teams, while IBM Watson Studio caters to enterprise solutions with graphical tools for quick model creation.
Each platform offers distinct advantages in user experience, from Google’s open-source Jupyter Lab interface to Azure’s tight Microsoft ecosystem integration. Your choice will depend on your specific needs and preferences in the cloud-based machine learning landscape.
Key Features Comparison
When comparing key features of top cloud-based machine learning platforms, it’s essential to understand their unique strengths.
Google Cloud AI Platform offers extensive support for the machine learning lifecycle, including data labeling, AutoML, and robust model deployment options. It integrates seamlessly with popular frameworks like TensorFlow and Scikit-learn.
AWS SageMaker provides a fully managed service with built-in Jupyter notebooks, pre-built algorithms, and distributed training capabilities.
Microsoft Azure Machine Learning excels in end-to-end lifecycle management, supporting various programming languages and offering automated ML capabilities. It also integrates well with Azure DevOps for streamlined workflows.
Databricks Unified Data Analytics stands out for its Apache Spark-based analytics, featuring collaborative notebooks and efficient data processing through integration with various data sources.
IBM Watson Studio offers a collaborative environment for data scientists, supports multiple data sources, and includes AutoAI for automated model building.
Each platform has its strengths in different areas of the machine learning lifecycle.
Google Cloud and Azure focus on extensive lifecycle support, while AWS SageMaker emphasizes managed services.
Databricks excels in data processing and analytics, and IBM Watson Studio shines in collaboration and automated model building.
Consider your specific needs when choosing the best platform for your projects.
Industry-Specific ML Solutions
While cloud-based machine learning platforms offer versatile solutions, many providers have developed industry-specific offerings to address unique sectoral challenges.
In healthcare, IBM Watson Health leverages AI-powered solutions for patient data analysis and personalized treatment recommendations.
DataRobot caters to the finance sector with automated machine learning tools for credit scoring and fraud detection, reducing operational risk.
Google Cloud AI Platform enables marketing teams to optimize ad spending through predictive analytics and advanced data analysis.
For retailers, AWS SageMaker provides machine learning capabilities for inventory management and customer behavior analysis.
In manufacturing, Microsoft Azure Machine Learning supports predictive maintenance solutions to reduce downtime and improve efficiency.
These industry-specific machine learning solutions offer:
- Tailored algorithms designed for sector-specific problems
- Pre-trained models using relevant industry data
- Customized interfaces and workflows aligned with industry practices
Performance and Scalability Analysis
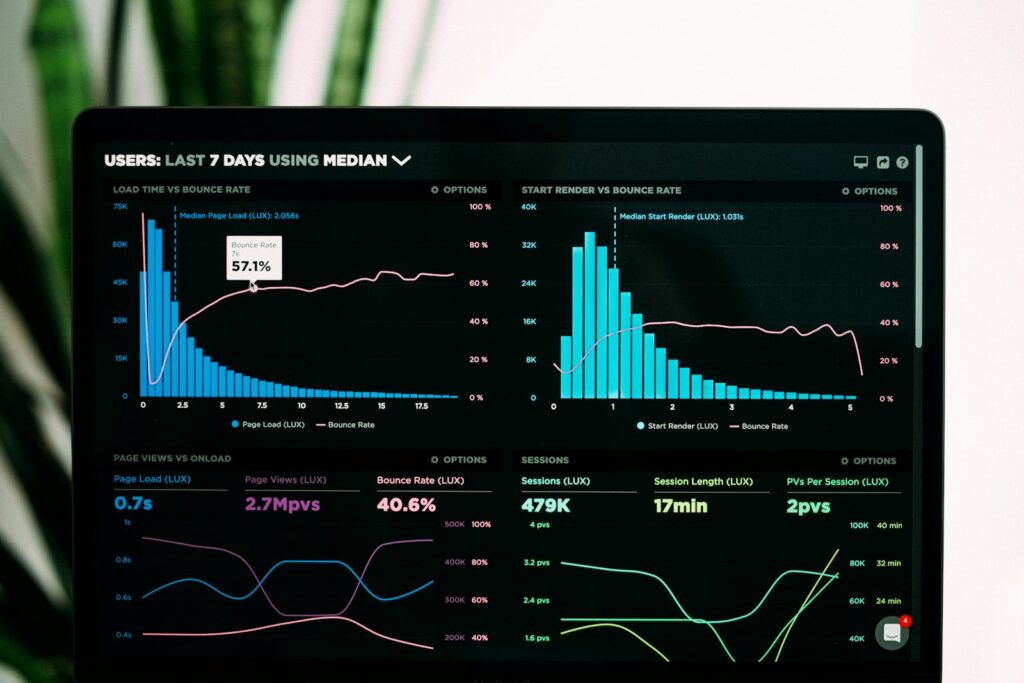
Analyzing performance and scalability is essential when selecting a cloud-based machine learning platform. When evaluating these platforms, you’ll find that each offers unique advantages for handling large datasets and complex model training.
Google Cloud AI Platform stands out with its virtually unlimited scalability and specialized TPUs for accelerating machine learning workflows.
AWS SageMaker provides flexible instance types, allowing you to scale your model training and inference workloads based on your computational needs.
Microsoft Azure Machine Learning offers various VM sizes and automated scaling features for efficient resource management.
For those working with massive datasets, Databricks Unified Analytics’ Lakehouse architecture combines the benefits of data warehouses and data lakes, enabling seamless scalability across multiple cloud environments.
IBM Watson Studio excels in distributed training, maximizing performance for large-scale data processing and model deployment.
When choosing a platform, consider your specific requirements for performance and scalability.
AWS SageMaker’s pay-per-use model can be cost-effective for varying workloads, while Google Cloud’s TPUs might be ideal for intensive deep learning tasks.
Evaluate each platform’s ability to handle your expected data volumes and computational demands to guarantee peak performance as your machine learning projects grow.
Pricing Models and Cost Efficiency
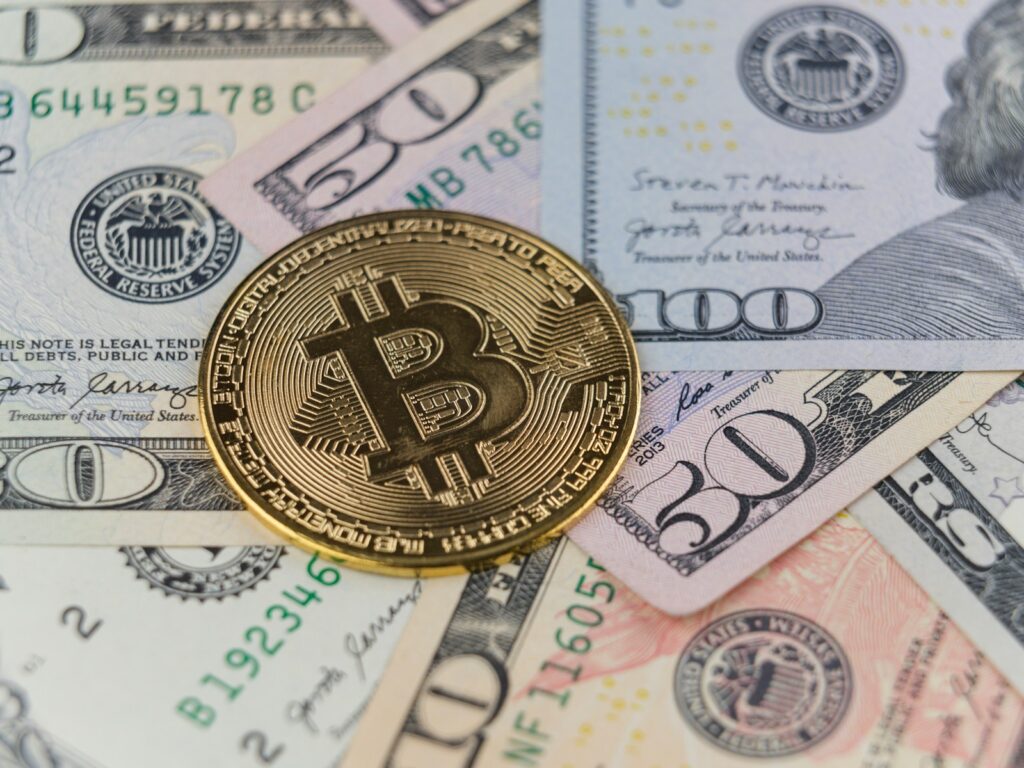
With respect to pricing models and cost efficiency, cloud-based machine learning platforms offer a wide range of options to suit different budgets and usage patterns.
You’ll find that pricing structures vary considerably across providers, from pay-per-use models to annual subscriptions. For instance, Google Cloud AI Platform charges $0.19/hour and offers a $300 credit for new users, while AWS SageMaker bases its pricing on computation hours with additional costs for data storage.
When considering cost efficiency, it’s vital to evaluate your specific needs and usage patterns. Here’s what you should keep in mind:
- Hidden costs can lead to budget overruns, especially for small to mid-sized businesses.
- Enterprise-focused platforms like DataRobot come with premium pricing, starting at $100,000 annually.
- Tiered pricing structures, such as those offered by Akkio, cater to non-technical users and integrate with big data tools.
Be aware that extensive usage of cloud-based machine learning platforms can result in higher expenses due to data storage and additional features.
Careful budgeting is essential to avoid unexpected costs and guarantee you’re getting the most value from your chosen platform.
Integration and Compatibility Considerations
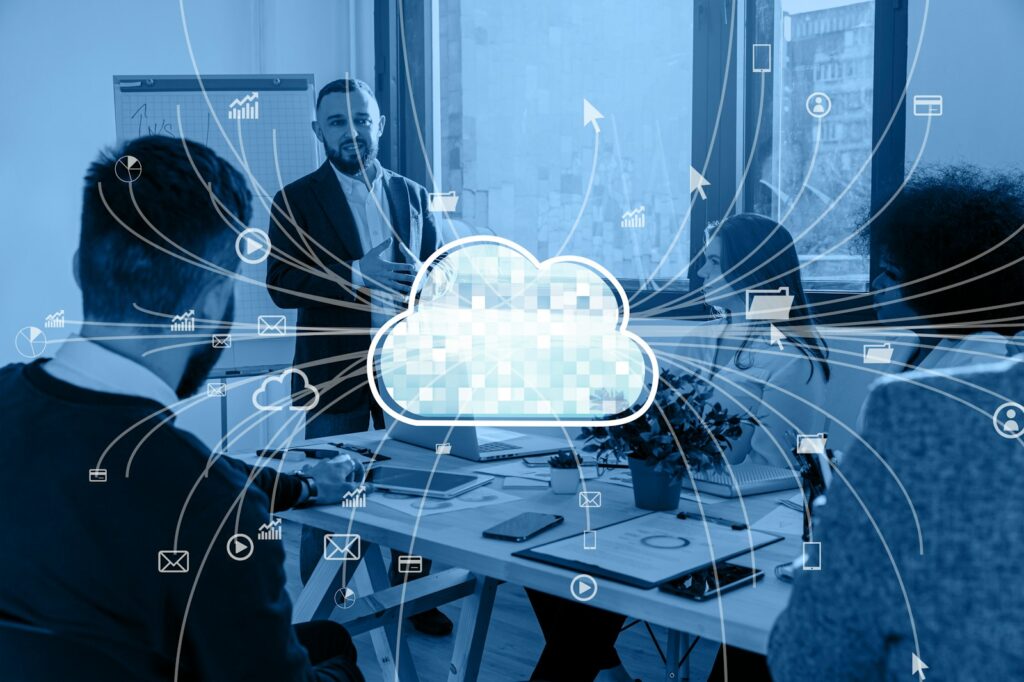
Beyond cost considerations, you’ll need to evaluate how well a cloud-based machine learning platform integrates with your existing systems and workflows. When selecting a platform, compatibility with your current infrastructure is vital.
Platforms like AWS SageMaker and Google Cloud AI offer seamless integration with their respective cloud services, which can be a significant advantage if you’re already using these ecosystems.
Consider the programming languages and frameworks supported by each platform. Microsoft Azure Machine Learning, for instance, allows data scientists to work with Python, R, and Java, providing flexibility in development.
API accessibility is another key factor; platforms like DataRobot and H2O.ai offer robust APIs to integrate machine learning capabilities into your existing applications.
Don’t overlook the importance of connecting to various data sources. Oracle AI Platform Cloud Service integrates deeply with Oracle databases, while Databricks supports multiple data lakes and warehouses.
For team-based projects, collaboration features are essential. IBM Watson Studio and Google Cloud AI Platform facilitate easy sharing and version control among team members working on machine learning models.
User Experience and Learning Curve
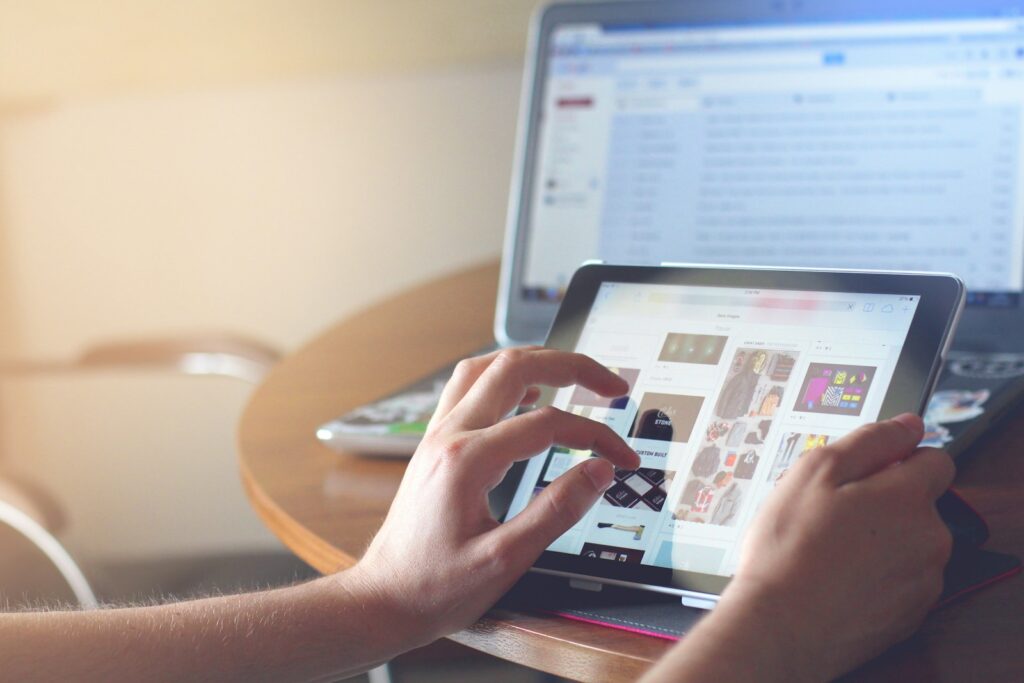
The user experience and learning curve of cloud-based machine learning platforms play an essential role in their adoption and effectiveness. Many platforms offer user-friendly interfaces that notably reduce the learning curve for non-technical users. These interfaces, combined with thorough documentation and tutorials, enable a broader range of professionals to engage with machine learning models.
Automated machine learning features, like those offered by DataRobot, streamline the process for users with limited expertise. This approach allows you to develop and deploy models without deep technical knowledge, enhancing ease of use. Additionally, platforms like AWS SageMaker provide built-in Jupyter notebooks, allowing you to leverage familiar coding environments for experimentation.
To paint a picture of the user experience benefits:
- User-friendly interfaces reduce the barrier to entry for non-data scientists
- Extensive training resources support continuous learning and skill development
- Well-designed onboarding processes decrease time to proficiency
The focus on user experience in cloud-based machine learning platforms has led to notable improvements in accessibility.
Security and Compliance Measures
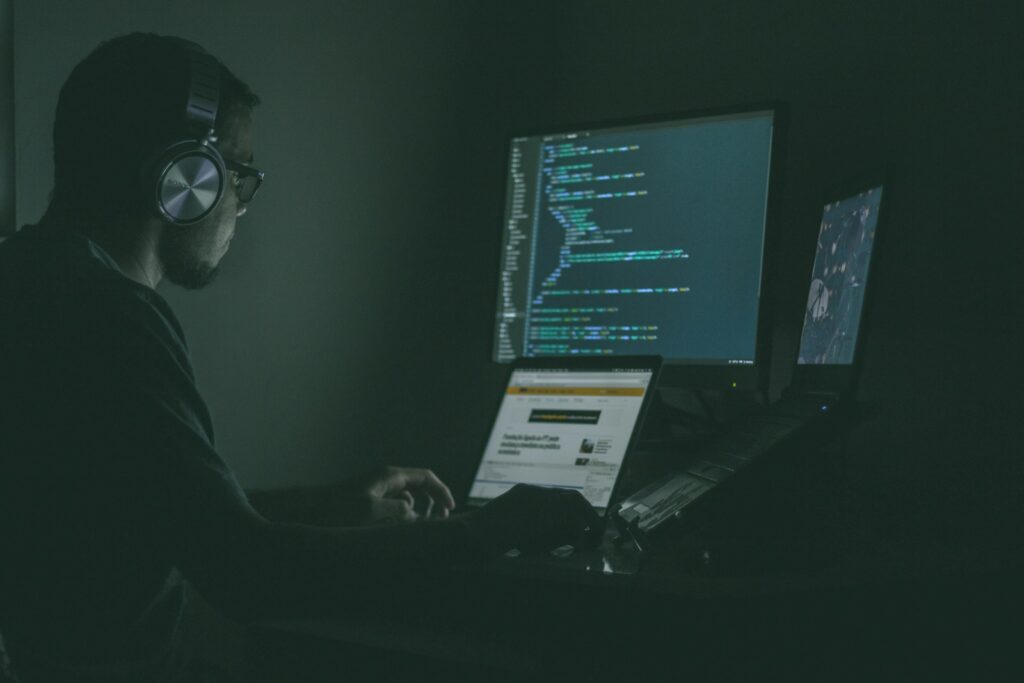
You’ll find that cloud-based machine learning platforms prioritize security and compliance through robust measures.
They implement strong data encryption protocols for information in transit and at rest, while adhering to regulatory compliance standards like GDPR, HIPAA, and CCPA.
Additionally, these platforms offer advanced access control mechanisms, allowing you to manage user permissions and monitor interactions with sensitive data effectively.
Data Encryption Protocols
In today’s data-driven landscape, robust encryption protocols form the backbone of security and compliance measures for cloud-based machine learning platforms.
You’ll find that these platforms implement stringent data encryption protocols to safeguard sensitive information during storage, transmission, and processing. The widely recognized AES standard, with key lengths of 128, 192, or 256 bits, is often employed to guarantee data confidentiality and integrity.
To maintain compliance with regulations like GDPR and HIPAA, cloud ML platforms typically utilize end-to-end encryption. This approach protects your data at every stage, from rest to transit and during processing.
It’s essential to choose a platform that adheres to these security standards and conducts regular audits to identify potential vulnerabilities.
When evaluating cloud-based machine learning platforms, consider the following:
- Implementation of AES encryption with appropriate key lengths
- End-to-end encryption capabilities for thorough data protection
- Compliance with relevant regulations such as GDPR and HIPAA
Regulatory Compliance Standards
Regulatory compliance juggernauts like GDPR and HIPAA shape the security landscape for cloud-based machine learning platforms.
You’ll find that these platforms must adhere to strict standards to guarantee data privacy and security for sensitive information. Many leading cloud ML platforms, such as AWS and Azure, offer built-in compliance certifications to help you meet industry standards and regulatory requirements.
To maintain regulatory compliance, you’ll see platforms implementing robust security measures. These include data encryption protocols, access controls, and audit logs to protect against unauthorized access and data breaches.
You’ll also find tools for data governance and management, which assist you in maintaining compliance throughout the machine learning lifecycle.
As you evaluate cloud ML platforms, consider their approach to regulatory compliance. Look for platforms that conduct regular security assessments and updates to adapt to evolving regulatory landscapes and emerging security threats.
Access Control Mechanisms
Access control mechanisms serve as the gatekeepers of cloud-based machine learning platforms, guaranteeing that only authorized users can interact with sensitive data and models. These platforms implement robust security measures to protect your valuable assets and maintain compliance with industry standards.
Role-based access control (RBAC) is a cornerstone of cloud ML platform security, aligning user permissions with job functions. This approach minimizes the risk of unauthorized access and helps maintain data privacy. Many platforms, including AWS SageMaker and Microsoft Azure Machine Learning, also incorporate multi-factor authentication (MFA) for an added layer of security.
To further enhance protection, cloud ML platforms employ:
- Data encryption at rest and in transit
- Compliance tools for GDPR, HIPAA, and PCI-DSS requirements
- Logging and monitoring features to track user activities and model changes
These access control mechanisms work in tandem to create a secure environment for your machine learning projects. By leveraging built-in tools and following best practices, you’ll be able to maintain the confidentiality and integrity of your data while meeting regulatory requirements.
The thorough security measures implemented by leading cloud ML platforms guarantee that you can focus on developing innovative models without compromising on data protection.
Conclusion
You’ve explored the top cloud-based ML platforms, each offering unique strengths. Consider your specific needs when choosing, weighing factors like performance, pricing, and integration. Don’t forget to evaluate user experience and security measures. While giants like Google, AWS, and Microsoft dominate, smaller players may offer specialized solutions. Ultimately, the best platform for you will align with your organization’s goals, technical requirements, and budget constraints. Take your time to make an informed decision.